AI and Automation: Revolutionizing Signal Detection in Drug Safety
- Chaitali Gaikwad
- May 14
- 3 min read
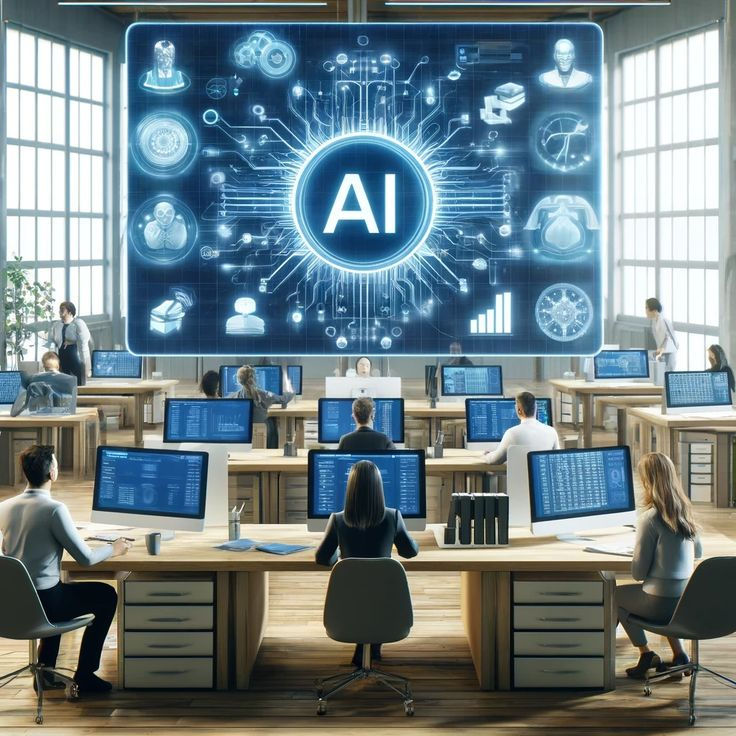
In the realm of pharmacovigilance, the timely and accurate detection of adverse drug reactions (ADRs) is paramount to ensuring patient safety. Traditionally, signal detection—the process of identifying potential safety concerns from vast datasets—has been a labor-intensive and time-consuming endeavor. However, the advent of Artificial Intelligence (AI) and automation is transforming this landscape, offering unprecedented efficiency and precision in monitoring drug safety.
Understanding Signal Detection in Pharmacovigilance
Signal detection involves the identification of new or known adverse events that may be associated with a drug. This process relies on analyzing various data sources, including spontaneous reporting systems, clinical trials, electronic health records (EHRs), and scientific literature. The goal is to uncover patterns or "signals" that warrant further investigation to confirm potential risks associated with a medication.
Challenges in Traditional Signal Detection
Traditional methods of signal detection face several challenges:
Volume of Data: The sheer amount of data from diverse sources can overwhelm manual analysis.
Data Complexity: Unstructured data, such as free-text clinical notes, complicate the extraction of meaningful information.
Latency: Delays in identifying signals can result in prolonged exposure of patients to potential risks.
Resource Intensive: Manual processes require significant human resources and are prone to inconsistencies.
These challenges underscore the need for more efficient and accurate methods of signal detection.
The Role of AI and Automation in Signal Detection
AI and automation offer solutions to the limitations of traditional signal detection methods:
1. Data Integration and Processing
AI algorithms can seamlessly integrate and process data from multiple sources, including EHRs, social media, and scientific literature. Natural Language Processing (NLP) techniques enable the extraction of relevant information from unstructured text, facilitating comprehensive analysis.
2. Pattern Recognition and Predictive Analytics
Machine Learning (ML) models can identify complex patterns and correlations within datasets, enabling the early detection of potential safety signals. These models can also predict future adverse events based on historical data, allowing for proactive risk management.
3. Real-Time Monitoring
Automated systems can continuously monitor data streams, providing real-time alerts for emerging safety concerns. This immediacy enhances the responsiveness of pharmacovigilance activities.
4. Resource Optimization
By automating routine tasks, AI reduces the burden on human analysts, allowing them to focus on more complex evaluations. This optimization leads to cost savings and improved efficiency.
Case Studies: AI in Action
Case Study 1: Enhancing Signal Detection with Machine Learning
A pilot study applied ML models to detect potential safety signals for two marketed products. The model demonstrated the capability to identify safety signals earlier than traditional methods, showcasing the potential of AI to augment pharmacovigilance efforts .
Case Study 2: AI-Driven Pharmacovigilance Platforms
Companies like PubHive have developed AI-powered pharmacovigilance software that automates signal detection, accelerates safety monitoring, and ensures compliance with regulatory requirements .
Regulatory Considerations
The integration of AI into pharmacovigilance must align with regulatory standards. Agencies like the FDA and EMA are increasingly recognizing the role of AI in drug safety monitoring. However, they emphasize the importance of transparency, validation, and accountability in AI applications to ensure patient safety.
Future Perspectives
The future of AI in signal detection is promising, with ongoing advancements in technology:
Advanced NLP: Improved NLP models will enhance the extraction of insights from unstructured data sources.
Deep Learning: Deep learning techniques will further refine pattern recognition capabilities.
Global Collaboration: Shared AI platforms can facilitate international collaboration in pharmacovigilance efforts.
Conclusion
AI and automation are revolutionizing signal detection in drug safety, offering tools that enhance the speed, accuracy, and efficiency of pharmacovigilance activities. By embracing these technologies, the pharmaceutical industry can better protect patients and respond swiftly to emerging safety concerns.
Request a live Demo!
Comments