AI-Powered Case Intake: Faster, More Accurate, and More Efficient
- Chaitali Gaikwad
- 4 days ago
- 4 min read
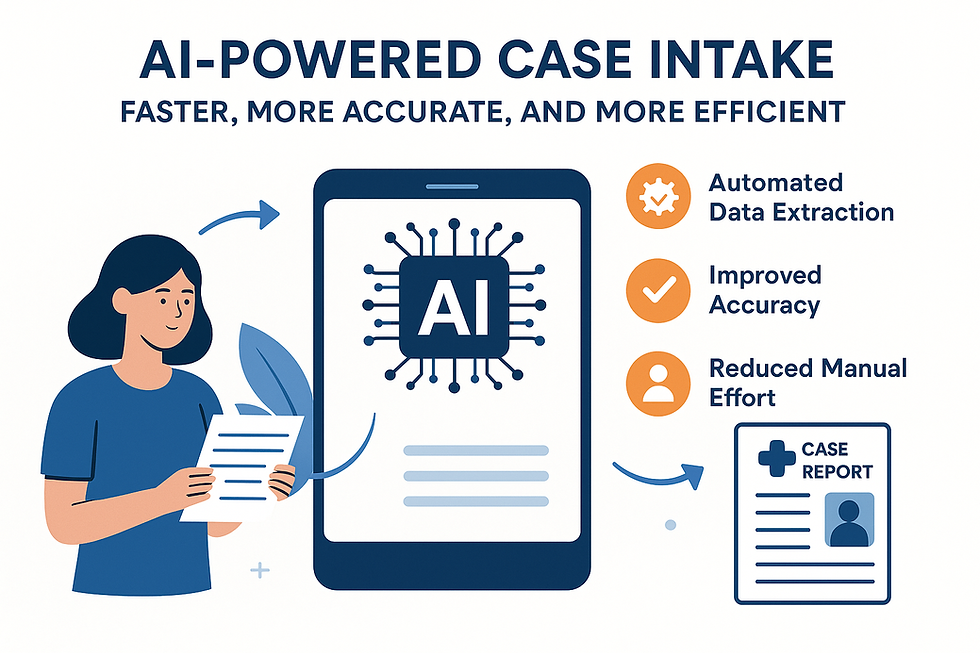
In the complex world of pharmacovigilance and healthcare, case intake represents the first critical step in ensuring patient safety. Traditionally, this process has been manual, time-consuming, and prone to human error. However, with the rise of artificial intelligence (AI), organizations are beginning to revolutionize case intake with greater speed, precision, and scalability. AI-powered case intake systems are not only streamlining operations but also enhancing data quality, improving compliance, and ultimately safeguarding public health.
This blog explores how AI is transforming the case intake process—making it faster, more
accurate, and significantly more efficient.
Understanding Case Intake in Pharmacovigilance
Case intake refers to the collection, documentation, and initial processing of individual case safety reports (ICSRs), which detail adverse events (AEs) or other safety information related to a medicinal product. This is a foundational activity in pharmacovigilance, as accurate and timely reporting is crucial for detecting potential safety signals and fulfilling regulatory obligations.
Traditional case intake involves:
Receiving data from various sources (emails, call centers, literature, EHRs)
Manually extracting relevant information (e.g., patient demographics, drug info, event details)
Validating and formatting data according to regulatory standards
Routing reports for further assessment or submission
This labor-intensive workflow can result in bottlenecks, delays, and inconsistencies—especially as report volumes continue to grow.
Challenges of Traditional Case Intake
Despite its importance, the conventional case intake process faces numerous obstacles:
High Manual EffortStaff must read and interpret unstructured documents, identify key data points, and enter them into safety databases. This consumes significant time and resources.
Inconsistency and ErrorsHuman interpretation of medical and safety information can vary. Errors in data entry or oversight in critical information can compromise patient safety and compliance.
Delayed ReportingSlow intake leads to delayed analysis and signal detection. Regulatory deadlines may be missed, risking fines or reputational damage.
Volume OverloadAs digital health expands, the volume of ICSRs increases exponentially. Manual processes are not scalable enough to handle this influx.
Multilingual ComplexityGlobal pharmaceutical companies receive safety reports in multiple languages, requiring translation and interpretation across diverse data formats.
The AI Advantage in Case Intake
AI technologies, particularly natural language processing (NLP), machine learning (ML), and robotic process automation (RPA), are now being used to transform case intake. Here's how AI changes the game:
1. Automated Data Extraction
AI systems can read and interpret unstructured documents such as emails, PDFs, medical records, or literature articles. Using NLP, they identify and extract key fields like patient details, adverse event descriptions, suspect drug information, and dates.
Benefit: Speeds up data collection and eliminates human error in identifying relevant data.
2. Faster Case Processing
Once the information is extracted, ML models classify and validate it automatically. They can determine seriousness, expectedness, and causality, and assign the case to the correct workflow.
Benefit: Enables real-time intake and triage, reducing turnaround time from hours to minutes.
3. Improved Accuracy and Consistency
AI systems are trained on thousands of cases and follow a consistent logic path. This ensures uniform data capture and reduces variability caused by human interpretation.
Benefit: Enhances data quality, supporting more reliable signal detection and regulatory reporting.
4. Multilingual Capabilities
Advanced NLP models can interpret safety information in multiple languages and standardize it for further processing. This removes the need for manual translation or local staff intervention.
Benefit: Enables seamless global case intake operations across different regions.
5. Scalability
AI-powered platforms can process high volumes of reports 24/7 without fatigue, making them ideal for managing spikes in case load (e.g., during product launches or safety alerts).
Benefit: Ensures operational continuity and scalability without increasing headcount.
Real-World Applications and Use Cases
1. Pharmaceutical Companies
Global pharma organizations use AI-powered intake to process ICSRs received through call centers, field force, and spontaneous reports. They’ve reported up to 70% faster processing time and over 90% accuracy in data extraction.
2. CROs and BPOs
Contract research organizations adopt AI to scale pharmacovigilance services for multiple clients. It reduces turnaround time and enhances SLA adherence.
3. Regulatory Submissions
AI streamlines the intake-to-submission pipeline by ensuring clean, validated data ready for reporting to agencies like the FDA, EMA, and MHRA.
4. Post-Marketing Surveillance
Real-time AI intake improves post-marketing monitoring by capturing and triaging AEs quickly, especially from social media, online forums, or EHRs.
Implementation Considerations
While the benefits of AI-powered case intake are compelling, successful implementation requires strategic planning:
✔ Data Quality
AI models must be trained on clean, labeled datasets for optimal accuracy. Poor-quality data can lead to errors or bias.
✔ System Integration
AI tools must integrate with existing safety databases (e.g., Argus, ArisGlobal) and reporting systems for seamless workflow.
✔ Regulatory Alignment
The AI system must meet GxP standards and ensure data integrity, audit trails, and traceability.
✔ Human Oversight
Though AI handles the bulk of intake, human review is essential for edge cases, escalations, and continuous improvement of AI performance.
The Future of AI in Case Intake
As AI technology continues to mature, we can expect even more advanced features:
Predictive Intake: Anticipating incomplete cases or follow-up needs based on historical patterns
Conversational AI: Using voicebots or chatbots to collect AE reports directly from patients or HCPs
Self-Learning Models: Continual improvement through feedback loops and human-in-the-loop corrections
Cross-Source Correlation: Linking data from multiple intake channels (e.g., EHRs + social media + literature)
These innovations will make pharmacovigilance systems more proactive, intelligent, and patient-centric.
Conclusion
AI-powered case intake is no longer a futuristic vision—it’s a present-day necessity for organizations aiming to scale, comply, and innovate in drug safety. By automating and accelerating the case intake process, companies can significantly enhance data accuracy, operational efficiency, and patient safety outcomes.
As the volume and complexity of safety data continue to rise, adopting AI isn’t just a competitive edge—it’s a strategic imperative. Forward-thinking pharmacovigilance leaders who embrace AI today will be best positioned to meet tomorrow’s challenges with confidence.
Comments