AI for Local Literature Surveillance: Enhancing Drug Safety Monitoring
- Chaitali Gaikwad
- May 14
- 4 min read
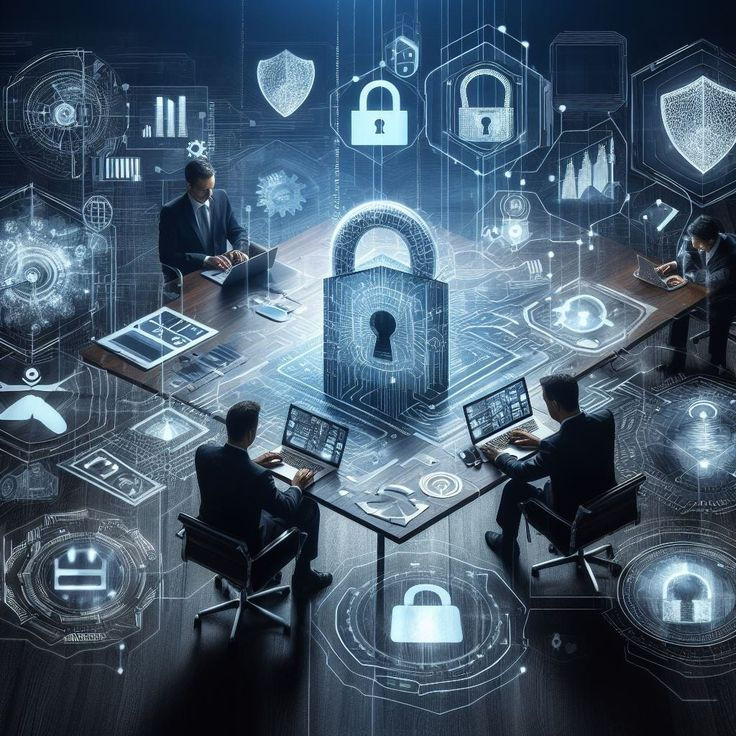
In the ever-evolving landscape of pharmacovigilance, the accurate and timely identification of adverse drug reactions (ADRs) remains a cornerstone of patient safety. Among the many sources of safety data, local literature is an essential but often underutilized component. Traditionally, manual methods of monitoring local journals and publications have been resource-intensive and inconsistent.
However, the advent of artificial intelligence (AI) has introduced transformative possibilities, especially in the realm of local literature surveillance. By leveraging AI, pharmaceutical companies, regulatory bodies, and contract research organizations (CROs) can now automate and optimize literature monitoring to ensure more effective and compliant pharmacovigilance operations.
Understanding Local Literature Surveillance
Local literature surveillance involves monitoring region-specific medical journals, publications, and news sources to identify mentions of adverse drug events, case studies, or drug-related safety issues. Unlike globally indexed literature, local publications may contain unique insights into regional ADR trends, off-label drug usage, and population-specific safety concerns. Hence, local literature plays a critical role in meeting the pharmacovigilance requirements of various health authorities, including the EMA, PMDA, and CDSCO.
Challenges in Traditional Local Literature Monitoring
Despite its importance, local literature surveillance faces several hurdles:
Language Barriers: Local publications are often in native languages, making it difficult for global teams to interpret.
Unstructured Data: Many local journals publish in formats that are not easily searchable or structured.
High Volume and Variability: The diversity and number of local sources make manual review time-consuming and error-prone.
Compliance Pressures: Regulatory agencies increasingly demand robust local literature monitoring, with non-compliance resulting in penalties or market access issues.
Enter Artificial Intelligence: A Paradigm Shift
AI technologies, including natural language processing (NLP), machine learning (ML), and optical character recognition (OCR), are redefining how local literature is accessed, reviewed, and analyzed. Here's how AI is revolutionizing this domain:
1. Automated Source Identification
AI systems can automatically identify and catalogue relevant local publications and data sources. This ensures comprehensive surveillance without the need for manual source discovery.
2. Multilingual Processing
Advanced NLP models are capable of processing texts in multiple languages, including those with complex grammar and regional dialects. This overcomes one of the primary challenges in local literature monitoring.
3. Text Mining and Entity Recognition
AI-driven text mining tools can extract key entities such as drug names, adverse events, patient demographics, and outcomes from unstructured narratives. This information is then categorized for further analysis.
4. Relevancy Scoring and Prioritization
Machine learning algorithms assess the clinical relevance of articles, enabling prioritization based on likelihood of containing reportable adverse events. This drastically reduces the manual workload.
5. Integration with Safety Databases
AI tools can seamlessly integrate extracted data into pharmacovigilance databases like Argus or ArisGlobal, facilitating streamlined case processing and regulatory reporting.
Benefits of AI-Driven Local Literature Surveillance
The incorporation of AI in local literature monitoring offers numerous benefits:
Improved Accuracy and Consistency: AI applies standardized rules, reducing human errors and variability in interpretation.
Faster Detection and Reporting: Real-time data extraction and processing enable quicker signal detection and case intake.
Cost Efficiency: Automation reduces the need for large manual review teams, cutting operational costs.
Scalability: AI can manage a growing number of sources and publications without additional human resources.
Regulatory Compliance: Structured audit trails and consistent processing enhance readiness for inspections and audits.
Real-World Applications and Case Studies
Case Study 1: Global Pharma Company
A major pharmaceutical firm implemented AI for monitoring over 1,000 local journals across Asia and Latin America. As a result, the company saw a 40% increase in identified reportable ICSRs and reduced case processing time by 60%.
Case Study 2: Regulatory Authority
A national drug regulator adopted an AI solution to track local news and publications for pharmacovigilance purposes. The tool flagged potential safety signals in near real-time, enhancing proactive risk management.
Case Study 3: Mid-sized CRO
A contract research organization used AI-powered NLP tools to streamline literature monitoring for multiple clients. This reduced turnaround times and improved client satisfaction while maintaining regulatory compliance.
Implementation Strategy: How to Get Started
Organizations looking to adopt AI for local literature surveillance should consider the following roadmap:
Needs Assessment: Identify specific goals such as language coverage, volume of sources, and integration requirements.
Vendor Evaluation: Assess AI vendors based on their pharmacovigilance expertise, NLP capabilities, and regulatory compliance features.
Pilot Programs: Begin with a small-scale pilot to evaluate performance and ROI.
Integration and Training: Work with IT and PV teams to integrate AI tools with existing systems and provide user training.
Continuous Optimization: Regularly review system outputs and update models based on feedback and new data.
Future Outlook: What Lies Ahead
The role of AI in pharmacovigilance is expanding rapidly. For local literature surveillance, we can expect the following advancements:
Voice and Image Recognition: Monitoring of podcasts, webinars, and video content for drug safety information.
Predictive Signal Detection: AI models that can predict potential safety issues before they become widespread.
Real-Time Collaboration: Cloud-based platforms that enable collaborative review and decision-making among global teams.
Conclusion
AI is reshaping the landscape of local literature surveillance by offering unparalleled speed, accuracy, and efficiency. As regulatory expectations grow and the volume of regional safety data expands, leveraging AI is no longer optional but essential. By automating literature review processes, organizations can not only ensure compliance but also enhance their ability to protect patient health. The future of pharmacovigilance will undoubtedly be driven by intelligent technologies, and local literature surveillance stands as a prime example of this transformation.
Yorumlar