Automating Local Literature Review: The Future of Pharmacovigilance
- Chaitali Gaikwad
- 1 day ago
- 4 min read
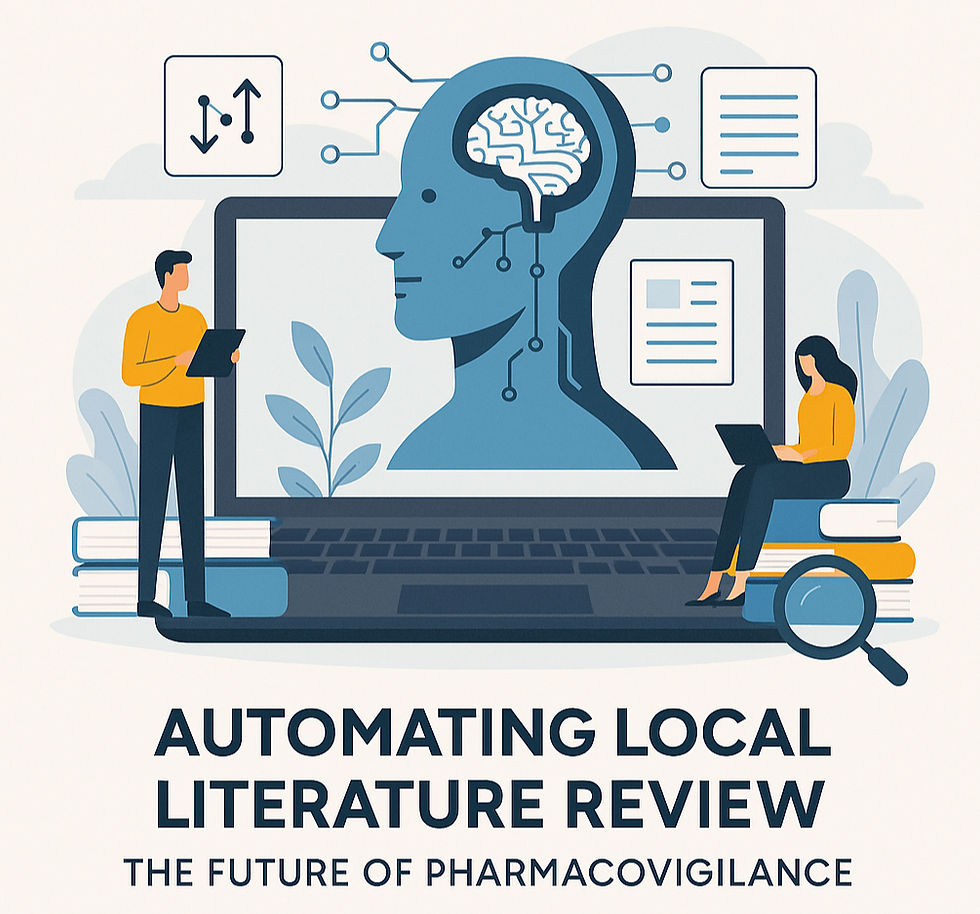
In an era where patient safety and regulatory compliance are paramount, pharmacovigilance (PV) has become a critical function for pharmaceutical companies, regulatory authorities, and healthcare providers. Among its many components, local literature review (LLR) plays a vital role in detecting adverse drug reactions (ADRs) and ensuring up-to-date drug safety information. However, the traditional approach to LLR is labor-intensive, time-consuming, and often inconsistent. Enter automation: a game-changer in how local literature is scanned, reviewed, and integrated into the broader pharmacovigilance framework.
What Is Local Literature Review (LLR)?
Local literature review is a mandated process under Good Pharmacovigilance Practices (GVP), particularly Module VI, which requires the systematic review of scientific and medical literature published in local journals to identify ICSRs (Individual Case Safety Reports), emerging safety signals, and risk information. The intent is to ensure comprehensive ADR surveillance beyond global databases and widely cited international journals.
LLR is crucial for:
Capturing ADRs reported in regional languages and lesser-known journals.
Complying with country-specific regulatory requirements.
Providing a holistic understanding of a drug’s safety profile in different populations.
The Challenges of Manual LLR
Despite its importance, LLR has traditionally been conducted manually by local affiliates or vendors. This manual process includes:
Identifying relevant local journals.
Accessing and downloading publications.
Screening for safety-relevant content.
Extracting and translating necessary data.
Submitting findings into global PV systems.
The manual nature of this work introduces several challenges:
Time and Resource Intensive: Reviewing hundreds of publications regularly requires considerable human effort.
Variability in Quality: Different reviewers may interpret content inconsistently, affecting data quality.
Delays in Detection: Manual reviews can result in slower identification of important safety information.
Language Barriers: Non-English publications require translation, increasing complexity and potential for errors.
Why Automation is the Answer
The digitization of healthcare data and advancements in artificial intelligence (AI), natural language processing (NLP), and machine learning (ML) have opened new doors for automating literature reviews. Automation in LLR involves using AI-powered tools that can scan, filter, interpret, and extract relevant safety data from a wide array of local publications efficiently and consistently.
Key Features of Automated LLR Systems
Journal Identification and Management:
Automated systems maintain updated libraries of relevant local journals.
They can dynamically add new journals as regulatory expectations evolve.
Text Mining and NLP:
NLP enables machines to understand human language, even in complex medical texts.
Systems can identify key terms, adverse events, drug names, and causality cues.
Multilingual Capabilities:
Advanced systems can handle multiple languages, reducing the need for human translation.
They can standardize terminologies using medical dictionaries like MedDRA.
Automated Filtering and Triage:
Not all articles contain relevant safety information.
AI models can filter out non-relevant articles and highlight those requiring human review.
Data Extraction and Structuring:
Once relevant articles are identified, systems can extract ICSR data fields such as patient demographics, drug details, event descriptions, and outcomes.
Integration with PV Systems:
Structured data can be seamlessly integrated into safety databases, expediting case processing and signal detection.
Benefits of Automating Local Literature Review
1. Improved Efficiency and Speed
Automated LLR dramatically reduces the time required to process literature, allowing for near real-time surveillance. What previously took weeks can now be done in days or even hours.
2. Scalability
As pharmaceutical companies expand into new markets, the number of local journals to review increases. Automation allows for scalable solutions that grow with organizational needs.
3. Consistency and Quality
AI-driven systems apply the same rules and logic across all reviews, minimizing human variability and increasing the reliability of the data collected.
4. Cost Savings
Reduced dependency on manual labor translates to significant cost savings over time. Automation also reduces the likelihood of regulatory penalties due to delayed or missed ADR reporting.
5. Enhanced Compliance
Automated tools often come with built-in audit trails and compliance tracking features, ensuring transparency and regulatory readiness.
Real-World Applications
Several organizations are already leveraging automation to optimize their LLR workflows. For instance:
Global Pharma Companies: Some have implemented AI tools that scan local journals in over 50 languages and automatically flag potential ICSRs for further human review.
National Regulatory Agencies: Agencies in countries like Japan and Germany have piloted AI models to assess the feasibility of national-level automated LLR.
CROs and Safety Vendors: Contract Research Organizations are increasingly offering automated LLR as part of their pharmacovigilance services, reducing turnaround times for their clients.
Challenges and Considerations
While automation offers many advantages, it also introduces new complexities:
Initial Setup Costs: Implementing AI and automation tools can be costly initially.
Data Access: Some local journals may not be digitally accessible or may require subscriptions.
Validation and Auditability: Systems must be validated and compliant with regulatory standards like GVP, FDA 21 CFR Part 11, and EU Annex 11.
Human Oversight: Despite automation, human pharmacovigilance professionals remain essential for oversight, validation, and interpretation of complex cases.
The Road Ahead
As technology continues to evolve, the future of automated LLR looks promising. We can expect:
Greater Adoption: As awareness grows, more companies and regulators will adopt automated LLR solutions.
Improved Algorithms: With continuous training, AI models will become more accurate and context-aware.
Global Standardization: Initiatives to create standard data formats and guidelines for automated LLR will support broader implementation.
Integration with Other PV Functions: LLR automation will be part of a larger AI-enabled pharmacovigilance ecosystem, integrating case processing, signal detection, and regulatory reporting.
Conclusion
Automating local literature review is no longer a futuristic concept—it is the present and future of pharmacovigilance. With the growing volume of data, increasing regulatory scrutiny, and the need for rapid responses, automation provides a viable, efficient, and compliant solution.
book your personalized Demo now!
Comments