The Impact of AI on Global Literature Review in Drug Safety
- Chaitali Gaikwad
- 4 days ago
- 3 min read
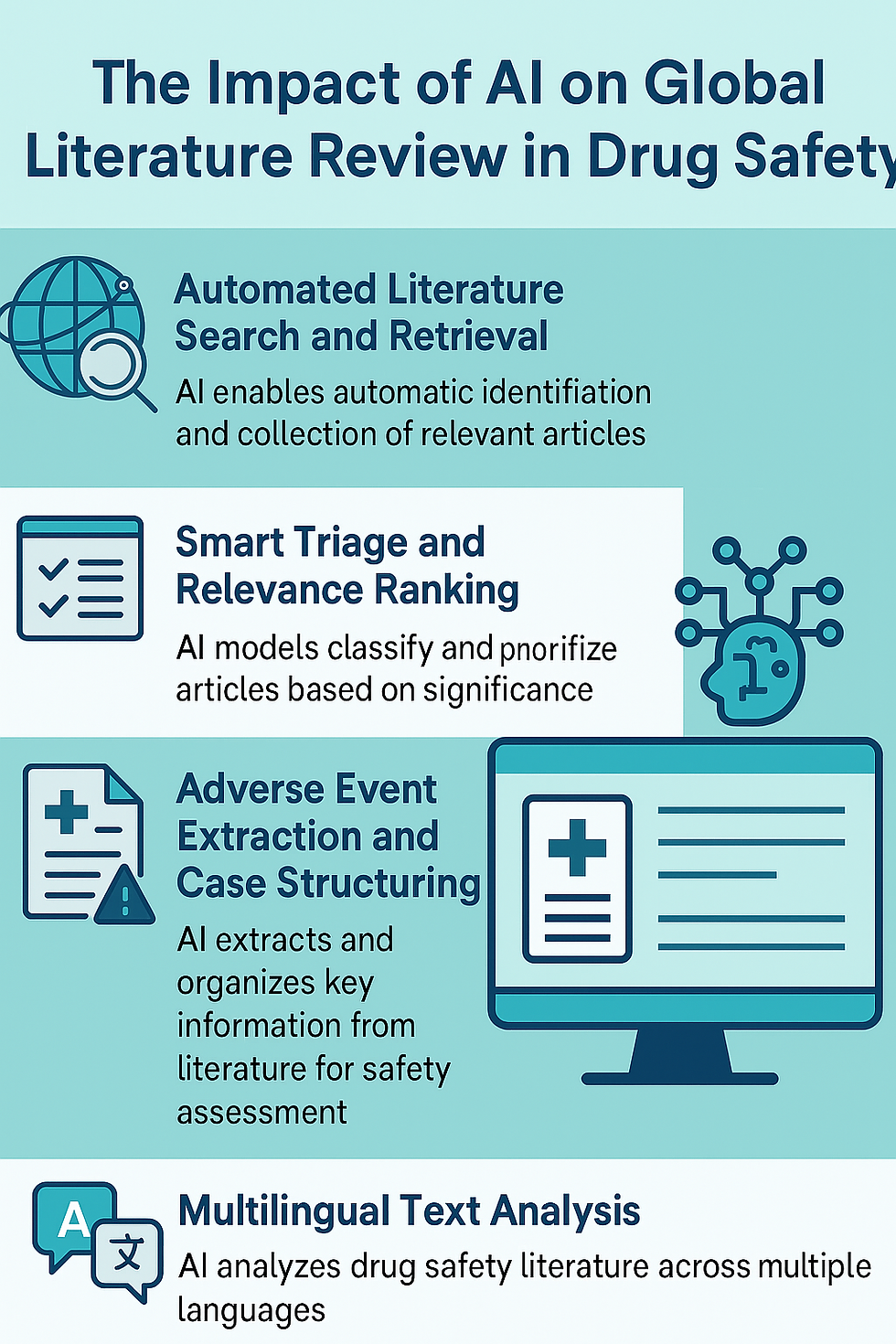
In the rapidly evolving world of pharmacovigilance, keeping up with scientific literature is essential for ensuring drug safety. Monitoring literature for case reports, safety signals, and emerging risks is not just a best practice—it’s a regulatory requirement enforced by authorities such as the EMA, FDA, and MHRA. But as the volume and complexity of global literature increases, traditional manual methods of literature review are struggling to keep pace.
Enter artificial intelligence (AI).
AI is revolutionizing the way pharmaceutical companies and regulatory agencies approach literature monitoring. By automating routine processes, enhancing accuracy, and enabling real-time surveillance, AI is transforming the global literature review into a faster, more efficient, and highly scalable operation. This blog explores how AI is reshaping global literature review in drug safety, the benefits it offers, and what the future holds.
Why Literature Review Is Critical in Pharmacovigilance
Scientific literature is one of the key pillars of post-marketing safety surveillance. It provides timely, peer-reviewed insights into drug-related adverse events (AEs), clinical trial outcomes, case studies, and observational data. Literature review serves multiple pharmacovigilance objectives:
Identification of adverse drug reactions (ADRs)
Detection of safety signals
Support for periodic safety update reports (PSURs)
Compliance with regulatory obligations
Understanding emerging usage patterns and risks
Global pharmaceutical companies must routinely monitor both indexed databases (like Embase, PubMed, and Medline) and local journals in native languages to ensure comprehensive coverage. However, with thousands of new articles published daily, manual methods can’t keep up.
Challenges of Traditional Literature Monitoring
Despite its importance, traditional literature review processes are often hampered by several challenges:
1. High Volume of Publications
An overwhelming number of journals publish health and drug-related content. Screening these manually is labor-intensive and time-consuming.
2. Language Barriers
Pharmaceutical companies with global operations must track literature in multiple languages. Limited multilingual capabilities among human reviewers can result in missed signals.
3. Inconsistent Triage
Different reviewers may interpret article relevance differently. This inconsistency affects the reproducibility and quality of results.
4. Slow Turnaround Times
Manual review processes are slow, increasing the risk of delayed case reporting and regulatory non-compliance.
5. Human Error
Fatigue, oversight, and variability in decision-making can lead to missed adverse events or inaccurate classifications.
These limitations not only increase compliance risk but also hinder timely identification of patient safety issues.
The Role of AI in Literature Monitoring
Artificial intelligence addresses many of these challenges by using technologies such as machine learning (ML), natural language processing (NLP), and semantic search to automate and enhance literature review workflows.
Let’s break down the ways AI is being used:
1. Automated Literature Search and Retrieval
AI-powered tools integrate with global literature databases and automatically retrieve articles based on predefined search criteria. These tools go beyond keyword matching by understanding the semantic context of queries, significantly improving the relevance of search results.
For example, AI can distinguish between:
“Pain” as a general symptom and “chest pain” as a critical adverse event.
“Drug A causes headache” vs. “Drug A treats headache.”
2. Smart Triage and Relevance Ranking
AI models, trained on annotated datasets, classify articles by relevance to pharmacovigilance:
Articles with potential Individual Case Safety Reports (ICSRs)
Reviews, editorials, and commentary
Duplicates or already known issues
These systems prioritize high-relevance articles for human review while deprioritizing non-relevant content.
This triage system helps reduce reviewer workload by up to 60%, allowing them to focus on meaningful content.
3. Multilingual Text Analysis
Global pharmacovigilance must account for non-English sources. AI with multilingual NLP capabilities can analyze articles in multiple languages, translate medical terms accurately, and extract key safety information across geographies.
For instance, AI can:
Translate and interpret Japanese, Spanish, and Mandarin articles.
Identify ADRs in non-English case reports.
Detect region-specific safety concerns.
4. Adverse Event Extraction and Case Structuring
AI tools automatically extract essential data from articles:
Patient demographics
Drug name and dosage
Adverse event details
Causality and outcomes
Using Named Entity Recognition (NER) and relationship mapping, these systems structure information in formats compatible with safety databases (e.g., Argus, ARISg).
This streamlines ICSR creation and reduces the need for manual data entry.
5. Continuous Learning and Customization
AI systems improve over time through machine learning. As users validate or correct classifications, the models learn from these interactions, enhancing future performance.
Pharma companies can also tailor AI systems to:
Focus on specific drug portfolios
Use proprietary medical dictionaries
Apply custom compliance rules
Conclusion
AI is redefining global literature review in pharmacovigilance by offering speed, scalability, and precision that manual processes cannot match. As the pharmaceutical industry continues to grapple with the challenges of rising data volumes and global regulatory expectations, AI presents a powerful solution to safeguard patient health while ensuring operational efficiency.
Book for a free demo today!
Organizations that embrace AI-driven literature review now will not only achieve compliance but also gain a strategic advantage in proactive risk management and innovation in drug safety.
Comments